Ccc Master Data Management
A refers to a data model, such as student or course, and is defined by the parties responsible for data governance. The data domain defines the and other attributes for that focus. Common examples of data domains are customers, students, employees, parts, and product orders. Data domains are typically created by the Data Governance Steward .
YOUnite allows the DGS to architect model for multiple domains as part of a single solution.
Once a version of a domain is created and its data records are loaded , it can be referenced by other data domains, adaptors, and API consumers as a source of truth. Domains have version numbers so that other domains, adaptors, and applications can bind to a specific domain and version . Data governance can designate data at specific adaptors as the master data and can control which zone has the appropriate ACLs to access and update it.
Strategic Data Management Board
This board is the central decision-making board in data governance that aligns and approves the data management strategy, annual objectives and budget. It also tracks the progress of the data management activities and resolves issues that had to be escalated. Oftentimes, the board consists of data definition owners from the business units and managers of the support functions as well as the Head of Data Management / Chief Data Officer. It is possible that these roles already meet in other boards or committees. If so, it should be considered whether an additional strategic data management board is really needed or whether the topics to be discussed can be integrated into the other boards or committees.
What Is Data Governance And Why Does It Matter
- Jack Vaughan,Senior News Writer
Data governance is the process of managing the availability, usability, integrity and security of the data in enterprise systems, based on internal data standards and policies that also control data usage. Effective data governance ensures that data is consistent and trustworthy and doesn’t get misused. It’s increasingly critical as organizations face new data privacy regulations and rely more and more on data analytics to help optimize operations and drive business decision-making.
A well-designed data governance program typically includes a governance team, a steering committee that acts as the governing body, and a group of data stewards. They work together to create the standards and policies for governing data, as well as implementation and enforcement procedures that are primarily carried out by the data stewards. Executives and other representatives from an organization’s business operations take part, in addition to the IT and data management teams.
Also Check: What Is The Return On Government Bonds
Successful Data Governance Requires Multilayer Management That Is Focused On Business But Spans Both Business And It
The Data Organizations Participants and Target Operating Model. Successful data governance requires multilayer management that is focused on business but spans both business and IT. It is typically built around chief data officers and their teamsthe only people specifically dedicated to data governanceplus a data governance council , data owners, data stewards, and data custodians.Notes:4Exact titles and granularity of roles of data governance differ from company to company, but they should result in the same thing: a stronger data foundation.
And what should data governances TOM be? We see four main organizational archetypes:
It is not uncommon for companies to begin by using a federated model with a doer CDO and, as the organization matures and builds its capabilities, to evolve to a CDO-as-facilitator model.
Over the past few years, data has been established as a fundamental source of business value. Companies compete in an environment characterized by enormousand growingdata sets, stringent data regulations, and frequent data-powered disruptions. In this context, data governanceand the resulting improvement of data qualityprovides a way to achieve not just short-term results but also to embed data in the organization and succeed in its data and analytics journey.
It just requires some attention and dedication to a data transformation. Any company can get it right.
What Is A Data Fabric

Data fabric is a design concept and architecture geared toward addressing the complexity of data management and minimizing disruption to data consumers while ensuring that any data on any platform from any location can be successfully combined, accessed, shared, and governed efficiently and effectively. A data fabric architecture is enabled by AI/ML-driven augmentation and automation, an intelligent metadata foundation, and a strong technology backbone .
Read Also: Us Government Cars For Sale
Retrieving Data Records With A Federated Domain: Data Record Assembly
Since YOUnite does not store the data records for federated data domains, it cannot return the entire data record in lists as it does with MDM_DATA_STORE domains. The first two requests below will return only the FDDPs and UUIDs for the data records in a domain. The third request shows to retrieve an assembled data record using a POST request. See the descriptions below for more details:
The FDDPs for data records or the default version of a domain can be retrieved with the following:
GET /drs?filters=name:< domain-name>
Retrieving the FDDPs for the data records for a given domain and version:
GET /drs?filters=name:< domain-name> ,version:< version>
Make a request for a specific federated data record. Since YOUnite must make requests to the adaptors where the data resides and assemble the data, the request payload must include a “callbackUrl” so YOUnite can deliver the payload ” rel=”nofollow”> http://youite.us/api). The POST request will return with a location that can be followed to check on the status of the request:
POST /drs/< dr-uuid> /assembler
What Is A Data Mesh
Data mesh focuses on organizational change enabling domain teams to own the delivery of data products with the understanding that the domain teams are closer to their data and thus understand their data better.
First defined by Zhamak Dehghani, a ThoughtWorks consultant who coined the term, data mesh is a type of data platform architecture that embraces the ubiquity of data in the enterprise by leveraging a domain-oriented, self-serve design. It enables data consumers to discover, understand, trust, and use data/data products to drive data-driven decisions and initiatives.
Just as engineering teams transitioned from monolithic applications to microservice architectures, data teams view data mesh as a prime opportunity to transition from monolithic data platforms to data microservices architecture.
Core to data mesh is the concept of breaking apart the monolithic architecture and monolithic kind of custodianship or ownership of the data around domains in the organization. Data warehouses and data lakes can still exist in the mesh architecture, but they become just another node in the mesh, rather than a centralized monolith.
Read Also: Government Jobs For History Majors
Data Fabric Vs Data Mesh: 3 Key Differences How They Help And Proven Benefits
Director, Platform Marketing
Monolithic, legacy architectures and centralized data platforms thwart business agility, making it difficult to quickly adjust to the constantly changing data landscape that demands new views, new aggregations, and new projections of the data . Both data fabric and data mesh architectures aim to abstract data management complexity and deliver data to the business with agility and scale.
Organizations relying on legacy architectures are struggling to scale data and analytics given the following challenges:
- Data proliferation and complexity Increasing data domains , data consumers, and data sources
- Lack of agility Inability to meet data demands in a timely manner
- Lack of collaboration Data developers siloed from the domains where the data originates or is used for decision making
- Lack of trust in data resulting from multiple copies of varying instances of the same data
These challenges hinder organizations from becoming truly data-driven and responding to business demands fast. Although these challenges are not entirely new to the data landscape, they have assumed greater importance as organizations strive to accelerate digital transformation.
What Is A Robust Data Governance Mandate
The mandate defines the authority granted to data management roles within the organization, and from this, the responsibility. This is particularly critical for the central data management team. Are they allowed to enforce the standards and guidelines for data creation and maintenance or are they dependent on the goodwill of the business units and support functions? The type of mandate has a significant influence on the overall impact of data management on the organization. Like the mandate of a project management office , four types of mandates can be distinguished.
Recommended Reading: Practice Test For Government Jobs
Some Additional Final Thoughts
DG 2.0As DG matures, there is a lot of talk about DG 2.0 What is really happening is maturity and addition of required capabilities. Personal opinionthere is no such thing as DG 2.0I do not see any new, unheard of capabilities that were not needed before. There is new technology to support DG. But that means new customers for DG, not DG 2.0.
Data DebtThis edition introduced the concept of data debt as a metric of sorts for DG. Briefly, if you make decisions without considering the impact on, or use of, data there are costs. The costs occur in the future via dealing with lack of consistency, errors, redundancy, etc. Like any other future obligations, there is interest to be paid. It gets more expensive to fix later than now.
Using data debt as a guidepost is effective. A major financial institution used data debt as a means to fortify DG oversight of its application development areas. We cannot afford more data debt from disjointed applications. A data glossary is to be maintained and followed to ensure consistency. No development is permitted that uses files, tables, or data sources that are not in the glossary and no development can be done without maintaining the glossary. The italics are the authors. This data debt-driven mandate worked, and the DG program got significant traction.
John Ladley, in, 2020
Measure Your Goals With Metrics
Lastly and certainly not least, a crucial data governance best practice is measuring your data governance program. Your team needs to evaluate the data governance programs progress and its impact on the rest of the organization.
This data governance best practice tends to be the most challenging for organizations, but it is an essential element to powering continuous improvement.
The first step is to understand and define what success looks like. Success at your organization will not look like success at another organization. Success will depend on what your organizations overall objectives are. Ask yourself and your teammates
- What does a successful data governance program look like?
- What does it mean to achieve Data Intelligence?
- How will we know when we achieved Data Intelligence?
Once, you can answer the above questions, you can identify your goals and evaluation criteria. Your key performance indicators should relate directly to your organizations objectives and strategies. Some areas to track are
These are just some of the data governance best practices that Data Intelligent organizations follow. And depending on the industry, there are different approaches. To learn more about data governance practices and how they generate positive business outcomes, check out these success stories.
The Data Intelligence company
We accelerate trusted business outcomes by connecting the right data, insights, algorithms and people for all Data Citizens.
Related resources
Recommended Reading: Federal Government Day Care Centers
Daily Operational And Regulatory Challenges
The following questions may seem familiar when looking at the daily operations of the data team and its stakeholders:
- Where can I find this data?
- What is this data for? What are the reports/metrics being generated using it?
- When was this data last refreshed?
- Who is the owner of this KPI or data ?
- How to get access to this data?
- Is the data trustworthy?
- What would the impact be if I make changes to some data KPI?
- Is it okay to share this data?
All the above questions can be broadly categorised as 1. Know your data: Understand and classify your data.2. Control your data: Apply flexible governance and security policies that dont hinder innovation.3. Integration across different applications: Simplify governance across the data landscape within one platform.
Communications Of The Acm
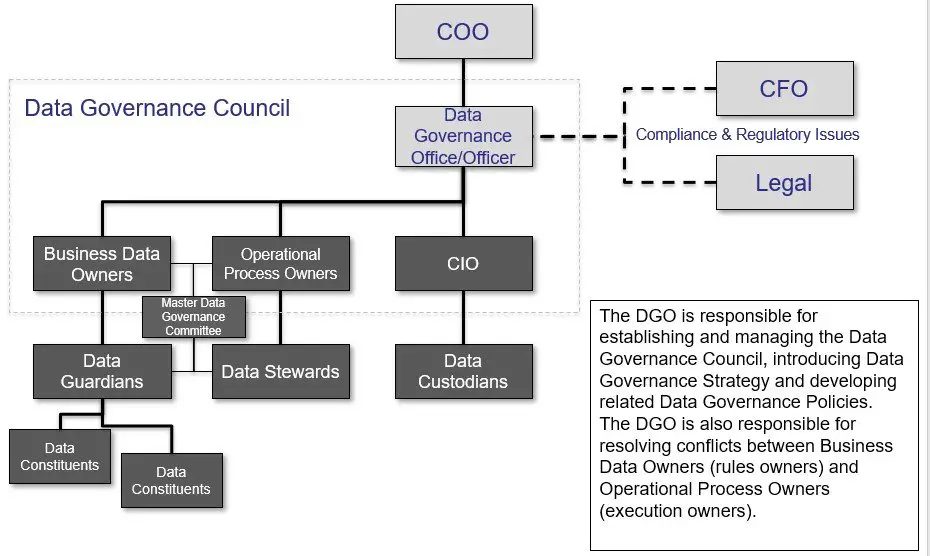
Organizations are becoming increasingly serious about the notion of “data as an asset” as they face increasing pressure for reporting a “single version of the truth.” In a 2006 survey of 359 North American organizations that had deployed business intelligence and analytic systems, a program for the governance of data was reported to be one of the five success “practices” for deriving business value from data assets. In light of the opportunities to leverage data assets as well ensure legislative compliance to mandates such as the Sarbanes-Oxley Act and Basel II, data governance has also recently been given significant prominence in practitioners’ conferences, such as TDWI World Conference and DAMA International Symposium.
The objective of this article is to provide an overall framework for data governance that can be used by researchers to focus on important data governance issues, and by practitioners to develop an effective data governance approach, strategy and design. Designing data governance requires stepping back from day-to-day decision making and focusing on identifying the fundamental decisions that need to be made and who should be making them. Based on Weill and Ross, we also differentiate between governance and management as follows:
- Governance refers to what decisions must be made to ensure effective management and use of IT and who makes the decisions .
- Management involves making and implementing decisions.
Read Also: How To Get A Free Computer From The Government
Data Governance Defines Who Does What In Data Management
The CC CDQ defines data governance as follows:
Data governance defines roles and assigns responsibilities for decision areas to these roles. It establishes organization-wide guidelines and standards, and it assures compliance with corporate strategy and laws governing data.
Best Practices: After defining responsibilities on paper, select and train the people who will take care of the data management activities on a day-to-day basis. It is recommended to select these individuals with utmost care. All too often, the people already overloaded with work also end up taking care of data. If that is the case, it will be impossible to achieve the agreed objectives, for instance, related to data quality.
Principles Underlying Data Governance
Two important design principles are fundamental for any data governance design:
- Enterprise-wide data governance requires collaboration between business, data and IT – Consequently, a federated approach is needed for enterprise-wide data governance. This implies that the roles and responsibilities can be assigned to employees who work in different parts of the enterprise.
- Data management and analytics roles facilitate the information supply chain – While the data management roles emphasize the provision of data for different business purposes, the analytics roles endeavor to deliver analytics products throughout the enterprise and integrate data across the business units. Both roles clearly depend on each other and facilitate information supply chains.
*Data definition includes business and quality rules, data access policies, data lifecycle and the conceptual data model. From the data point of view, it thus provides the input for the authorization concept, the risk management and compliance.
You May Like: Is The Government Shutdown Again
The Four Building Blocks Of Data Governance
Best practices create the optimal environment for successful data projects.
Data Structures. This is the starting point. Data structures help create an inventory and shared language around data. They include descriptions of the companys data, defined and classified in glossaries, domains, families, models, dictionaries, and flows. This isnt exactly new: companies have been using such tools for decades. More often than not, however, they are outdated, incomplete, and not fit for purpose.
The most important of these structures are data glossaries and data domains, which help define, organize, and assign the management of company data.
Data domains focus on where data resides and specify ownership of the data defined in the glossary. This high-level, unapologetic classification allows for no gaps and no overlaps. Achieving this is easier said than done. Some critical data is used in varying use cases and with different understandings of its meaning and purpose. It thus seems owned by many people or corporate entities. For example, who owns the customer data? The sales department? Marketing? Finance?
Challenges For A Successful Data Management
But how many of such data management programs actually become successful? Not many.
A company is recognized basedon direct customer-facing value propositions. Its success is measured upon product sales and customer engagement. So the profit thinking and focus on creating differentiation in terms of richer offering is always the priority. While data is at the core of most products, it is often the outer layer of application functionality and user experience that shadows the core.
Implementing data management organization-wide rarely becomes successful. Value stream leads need to focus on value propositions. Product owners need to focus on ways to improve customer experience. While the objective of data management is to make such things easier, it ends up being an overhead that slows them down and creates unnecessary dependencies.
Don’t Miss: California Government Tort Claim Form
It Governance As The Context For Data Governance
IT governance refers to who holds the decision rights and is held accountable for an organization’s decision-making about IT assets. In their IT governance framework, Weill and Ross propose that governance design includes five major decision domains: IT principles IT architecture IT infrastructure Business application needs and IT investment and prioritization. Although the five key decisions are interrelated, each of these decisions deals with a distinctive set of core issues. IT principles clarify the role that IT plays in the organization and drive the IT architecture decisions that establish the IT infrastructure. The organization’s IT infrastructure capabilities enable its business application needs, and the need for new IT applications can create new IT infrastructure requirements. IT investment and prioritization decisions are in turn shaped by the organization’s IT principles, architecture, infrastructure, and application needs.