Data Governance Maturity Models Explained
It is a good practice to assess the maturity of your organizations system periodically. Maturity is the quantification of an organizations ability and scope for improvement in a particular discipline.
A high level of maturity implies higher chances of improvement after the occurrence of an error or any incidence for that discipline.
These improvements could be either the quality or the use or implementation of the resources within the organization.
Data maturity models help companies understand their data capabilities, identify vulnerabilities, and know in which particular areas, employees need to be trained for improvement.
It also helps organizations compare their progress among their peers.
With maturity assessment, there is never a one model fits all situation. Although individual models for different organizations and vendors do exist, most follow the Capability Maturity Model method.
Here, we will go through two Data governance maturity models developed by two different vendors. Lets dive right in.
Data As A Business Asset
The path to effective organisational policy-driven data governance begins with recognising data as a valuable business asset and works towards building a framework that cultivates the value that it provides. It involves stepping back from the day-to-day decisions and processes in order to see the bigger picture and understand how poor data quality management leads directly or indirectly to lost revenue. An important aspect of this is to ensure that this outlook transcends departments and represents a truly organisation-wide change in attitude and processes, with responsibility and accountability effectively assigned.
Define Your Maturity Levels
For doing an information governance maturity assessment, you need to determine the maturity levels that makes most sense for you based on your program scope. The above maturity models often contain good descriptions of each level. As example, this is how the MIKE2 model describes the different information management maturity levels:
Don’t Miss: How Do I Get A Grant To Start A Trucking Company
The Importance Of A Data Management Maturity Model For Organizations
“Picking the right maturity model is one of the most critical decisions for an organizations data strategy.”
The Data Management Maturity Model provides guidelines to help organizations build, improve, and measure their enterprise data management capability. It is a consistent, organization-wide framework used to implement data management practices. This leads to data that is accurate, timely, and accessible across the entire organization. The DMMM follows data strategies, policies, and regulations that align with industry standards.
What does the DMMM provide?
The history of maturity model dates back to August 1986, when the Software Engineering Institute at Carnegie Mellon University, began with assistance from the MITRE Corporation to develop a maturity framework that would help organizations improve their software processes.
According to the results, 50% of respondents use DCAM v2 , whereas 17% use DAMA, CMMI custom maturity models. DCAM v2 is clearly a popular choice, however, it is essential to ensure that it is correctly implemented.
Reaching and Maintaining Maturity
Summary
What Are Data Governance Maturity Models
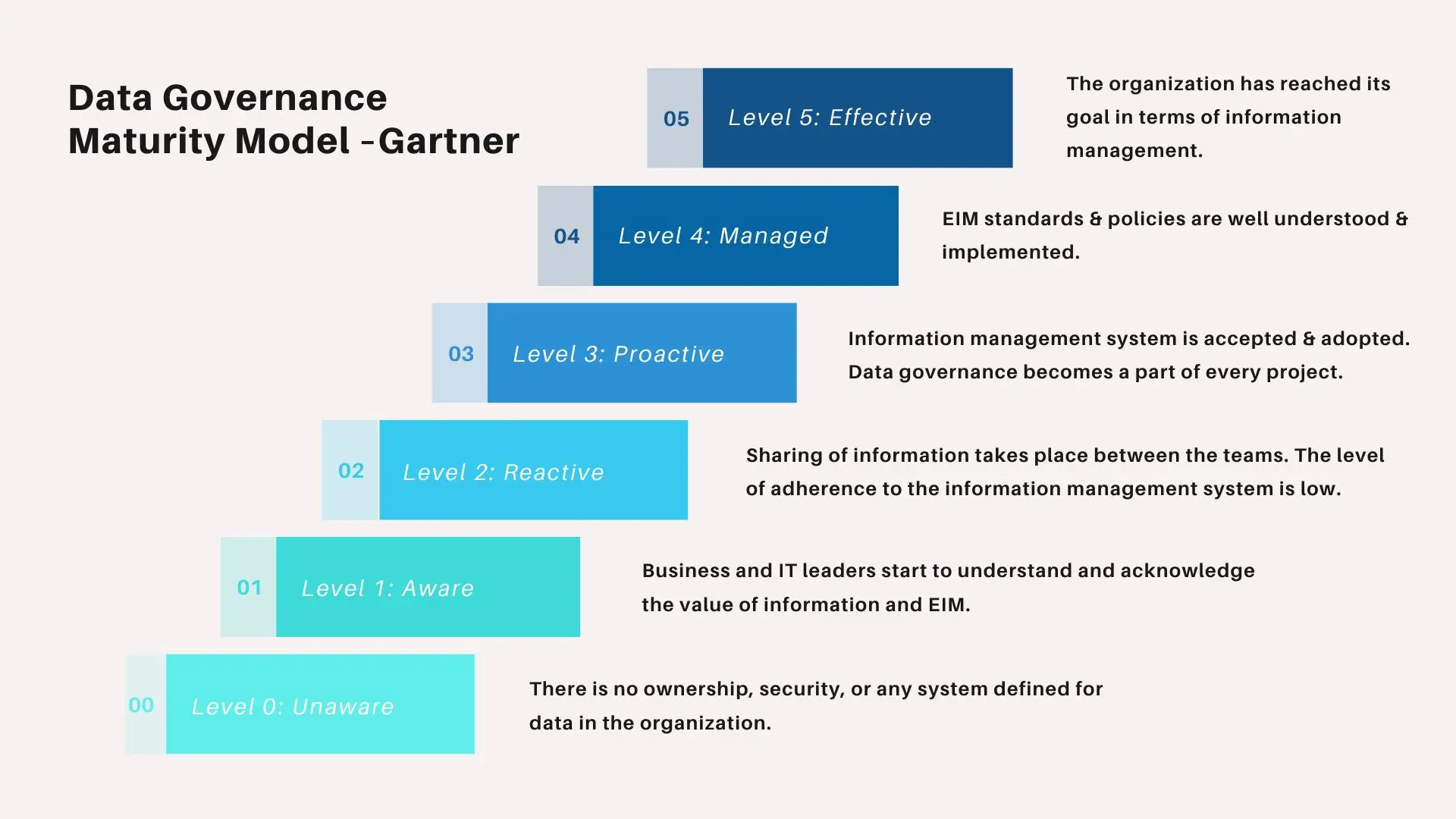
There are a number of different models that have been drafted and implemented by assorted movers and shakers within the data and analytics field. The purpose of these is to pinpoint the level of development an organisation is at in respect to a successful data governance framework and to highlight the steps that remain to be taken.
It is a good idea to have a general idea of how the journey to well governed data is understood and implemented by these organisations. An Executive Steering committee that is seriously committed to fundamental change is usually well advised to choose and aim to adhere to a well-structured framework with clear objectives and ways of gauging success.
The ARMA International model is based on the Generally Accepted Recordkeeping Principles: Accountability, Transparency, Integrity, Protection, Compliance, Availability, Retention and Disposition. An organisation is graded on each of these levels on a scale of competency starting with Level 1 which represents a baseline, to Level 5 which represents a data environment in which good governance is so integrated as to be routine.
These different roadmaps towards the process of data maturity and a data governance framework show that there is no one-size-fits-all solution within this space. Nevertheless, there are clear convergences across all of the highly structured and proven models.
Read Also: City Of Las Vegas Government Jobs
Useful And Practical Data
Proper implementation and practice of improved data standards impacts the entire enterprise-wide data lifecycle. Data is made to be useful and fit-for-purpose without going through unnecessary and time-consuming rounds of processing. Proper use of metadata, badging and rating of assets and visualisations ensures that everyone is clear on the usefulness and limitations of any given element. This is a good way to build organisation-wide confidence in data, which is a major step in achieving good Data Governance.
Understanding The Importance Of Data Governance Maturity Model
Data Governance is a paradigm for provisioning policies, best procedures, and practices on an enterprises structured or unstructured data here to help in managing the entire data lifecycle.
With the selection of a proper governance maturity model, a company covers every aspect of its data & information usage.
Data governance supports a company by magnifying its value, contemplating security-related risks, and cost-cutting trends.
A data governance maturity model is a framework for administrating and setting out the rules on how to treat data as an asset.
Data governance maturity ensures sound decision making and treats data within an organization as an asset from its inception to obliteration.
Data handling is done with sound data governance principles, deep-seated, and application. The model adaption is based on the established capability maturity model integration to the data governance context.
There is a general Capability Maturity Model with multiple data governance aspects that can be applied to many other processes as well. Maturity here means the degree of optimization done in the process by improving the given level/steps in the process.
The model improves the processes and structures of the governing data. CMM is based on a process model.
A process model is an arrangement of structured practices defining characteristics of productive methods, proven to be effective by experience.
Don’t Miss: City Of Las Vegas Government Jobs
Key Changes For Making A Transition To Diagnostic Analytics
Building a data-centered culture. Here, the main issues to overcome concern the company structure and culture. Breaking silos between departments and explaining the importance of analytics to employees would allow for further centralizing of analytics and making insights available to everyone. Given the company has a vision for further analytics growth, it must decide on the driver that will be promoting the data culture across the organization.
Introducing data engineering and data science expertise. Data engineering is required for building data infrastructure. At this point, organizations must either train existing engineers for data tasks or hire experienced ones. Also, the skill set of the business analyst is not enough for running complex analytics, so companies have to think about engaging data scientists.
Enhancing infrastructure. Companies at the descriptive analytics stage are still evolving and improving their data infrastructure. This entails testing and reiterating different warehouse designs, adding new sources of data, setting up ETL processes, and implementing BI across the organization.
Introducing systematic diagnostic analysis. Data analysts and data scientists may create some diagnostic and predictive reports on demand. For further transition, the diagnostic analysis must become systematic and be reflected both in processes and in at least partial automation of such work.
Build A Virtual Bi Team
Whats a virtual BI team? One that does work as needed.
A virtual team is organized around set goals, rather than set roles. Instead of a definite business intelligence department, which would take time and money to assemble, a virtual BI team is made up of stakeholders from across the companys pre-existing departments, on both the business and IT side.
Your virtual team exists to set up your BI strategy, then get it off the ground. Their purpose is to make sure your data and analytics program meets the needs of the companys departments, so that employees will be willing and able to act in a data-driven manner.
Where the training wheels come off: Your virtual team should not become a new center of power, or department. Instead, their goal should be to build a strategy that will encourage grass-roots interest and involvement in analytics.
To that end, when you go to shop for a business intelligence software program, make sure to look for one with self-service capabilities. Self-service means that any self in the company, regardless of technological knowledge, can use the program. Check YouTube, product forums, and customer reviews to find out if the program seems easy to use. If the software has a free trial version, download that and play around.
Also Check: City Of Las Vegas Government Jobs
Technological Perspective: First Attempts At Building Data Pipelines
Here, depending on the size and technological awareness of the company, data management can be conducted with the help of spreadsheets like Excel, simple enterprise resource systems and customer relationship management systems, reporting tools, etc. These tools, besides providing visualizations, can describe available data, for example, estimate the frequency distribution, detect extreme and average values, measure dispersions, and so on. Data is mostly analyzed inside its sources.
That said, technologies are underused. While allowing for collecting and organizing data, no deep investigation is available. So, analytics consumers dont get explanations or reasons for whats happening.
At this point, some organizations start transitioning to dedicated data infrastructure and try to centralize data collection. The key artifact of this centralization is data warehouses that can be created as part of an ETL data pipeline.
Check our detailed article to find out more about data engineering or watch an explainer video:
Data engineering, explained
In a nutshell, a data warehouse is a central repository where data from various data sources is organized and stored. Also, at the descriptive stage, the companies can start adopting business intelligence tools or dashboard interfaces to access the data centralized in a warehouse and explore it.
Data warehouse architecture
Which Data Governance Maturity Models Should You Use
Data governance maturity models help you measure and understand how well you manage your data. When you have a clear understanding of your data governance, youâre better able to understand how you are or are not meeting goals, what steps you need to take to improve data management overall, and how you need to tailor data approaches to each team and project.
There are almost as many data governance maturity models as there are use cases for data. If you already use a maturity model to measure your data capabilities, itâs best to adjust that framework and apply it to your data governance to keep things consistent.
If, however, youâre starting from the ground level, you can choose from one of the many maturity assessments published by reputable vendors, each of which features a slightly different emphasis:
- IBM& Deloitte focus on process characterization across projects and enterprise levels and use the CMMI Instituteâs Data Management Maturity model.
- DataFlux focuses on people, risk, process, reward, technology adoption, and business capabilities.
- Dattamza focuses on process, technology capabilities, managed risk, financials, and people management.
- EDM Council focuses on the maturity of an organizationâs structure, its policies and guidelines, and funding.
- Kalidofocuses on the value of data as an enterprise asset and the risks and rewards of how itâs managed.
You May Like: Goverment Jobs In Las Vegas
Data Governance Maturity Model
In the Implementation phase, we incrementally and iteratively apply the DGMM methodology.
DGMM is objectively assessing the capability of our clients to define, manage, and use their data in accordance with the data strategy, data principles, and data policies previously defined.
Incrementally, because a practical approach dictates that at first the focus should be on the critical data for the prioritized data domains, and then, as we progress, increase the scope to gradually include all data domains.Iteratively, because each time the scope is increased, the same standards have to be applied in order to achieve governance for the additional data domains in scope.
Follow-up audit and long-term coaching
By Level 5 The Process Of Digital Innovation Using Open Data Is Embedded Deeply Across The Entire Government
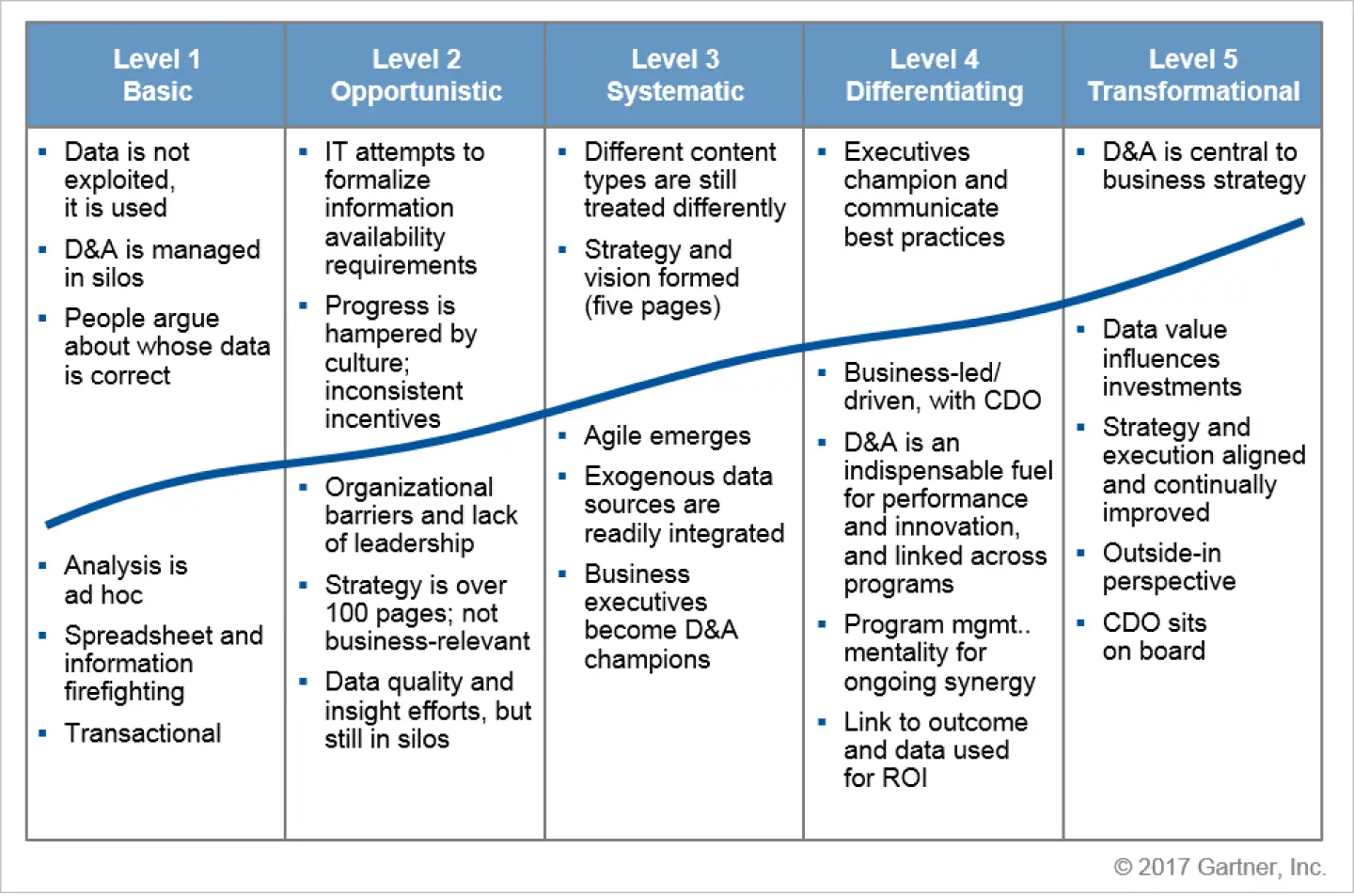
Speaking at Gartner Symposium/ITxpo in Barcelona, Andrea Di Maio managing vice president at Gartner, said that the vast majority of digital government strategies that Gartner evaluates are just modestly updated versions of a prior e-government strategy.
“We assess digital government maturity by examining the extent to which organizations use data effectively to redesign services and deliver new ones, as well as to transform and manage operations,” said Di Maio. Government leaders must replace their focus on services with a data-oriented mindset to truly transform government with new technologies such as artificial intelligence and the Internet of Things.
Di Maio recommended that government CIOs use Gartners 5 level maturity model to help plot their digital business strategy and communicate it to key policymakers and stakeholders.
Don’t Miss: Government Dental Grants For Seniors
Roles Around Data Governance
A few roles are key to the practice of data governance. Three roles ensure that standards are created and maintained over time, aiding in data compliance, security, data quality and automation goals.Chief data officer
Executive sponsors, such as chief data officers, signal the importance of a data governance program to the organization through its prioritization. These individuals are instrumental in the development of a cross-functional council, which usually sources members from various business units to represent the needs and concerns of different disciplines or product portfolios. This committee serves as a forum to communicate new data governance initiatives and assign responsibilities to achieve agreed upon timelines and outcomes.Data owners
These individuals are responsible for the state of the data. They are usually designated by the type of data that they manage, such as customer or financial data, and their role seeks to maintain data accuracy and usability. Common tasks include troubleshooting data issues, approving data definitions, and providing data recommendations, particularly as it relates to any regulatory requirements.Data stewards
What Is The Bi Maturity Model
The business intelligence maturity model is a five-level scale that tells you how mature your data and analytics strategy is. There are actually multiple business intelligence maturity models , but one of the top models is definitely Gartners.
Gartners business intelligence maturity model, from How to Accelerate Analytics Adoption When Business Intelligence Maturity Is Low
The low end of the business intelligence maturity model looks like this: your data is scattered across different, disconnected spreadsheets and documents. Employees may want information, but they ask for it in haphazard, one-off fashion. Also, no ones in charge of data governance.
The high end of the BI maturity model looks like this: you have a CDO , or at least someone in charge of wrangling your data. Your data is organized and accessible because your data sources are connected to a business intelligence software program. Employees check the data when they want to make any decision, so much so that data drives decision-making.
Recommended Reading: Governmentjobs.com Las Vegas
About George Firicanthe Course Teacher
George is a passionate advocate for the importance of data, a frequent conference speaker and a YouTuber, being ranked among Top 5 Global Thought Leaders and Influencers on Big Data, Digital Disruption and Top 15 on Innovation. His innovative approach to data governance received international recognition through several award-winning program and project implementations, including awards for developing a Data Governance and a Data Quality Program. George advises customer organizations on how to treat data as an asset, and he shares his practical takeaways on social media and various industry sites and publications.
George has been a Data Governance and Data Management practitioner for more than 10 years and he loves to create informative, practical and engaging educational content sharing it with individuals such as yourself. George is the proud founder of LightsOnData.com and co-host of the Lights On Data Show.
The Best Data Governance Maturity Model Course You Will Find
This online course is the smartest, most cost effective, and time saving option providing you the knowledge and understanding of data governance maturity models in order to lead you on the path on how to launch or improve your data governance program. It is developed to be a 1-stop-shop in getting:
- Explanation of the maturity modeling concepts, characteristics, benefits, pitfalls, and steps/ workflow
- Overview of well-known and not so well-known data governance and data management maturity models
- An engaging and fun learning experience with George Firican, your friendly data guy, who is great at turning dry knowledge into digestible and enjoyable bites
Plus this online course is the better way of learning:
- At your own pace
- From the comfort of your home or office, or anywhere you want to
- With unlimited access to the material
What would be the other options?
This online course gives you the know-how on numerous existing data governance and data management maturity models, and key learning and takeaways on how to select and use one with the least investment of time, money and energy. At the end of the course you will become knowledgeable in data governance maturity models and be better equipped to establish or improve your data governance program.
Bonus Content!
The course now includes a BONUS chapter covering a Data Lake and a Data Science maturity models.
You May Like: Trucking Business Grants
Heres What Youll Get When You Sign Up
This course has over three hours of content in the form of video lessons, supplemented by written content and other resources. Take it at your own pace and take advantage of the following benefits to learn all there is to know about Data Governance Maturity Models in order to establish or improve your data governance program.
Step-by-Step Instructions and Advice
In addition to the theoretical materials, the course contains the steps you should take in order to successfully select a model, and perform the assessment, to then analyze the results, and improve your data governance capabilities. These are all peppered in with plenty of advice learned from years of experience.
Overviews of Existing Models
Each of the Data Governance Maturity Models presented in this course is covered in its own lesson, going over the concepts, and the characteristics of each of its levels, phases, or milestones. It also goes into its domains and sub-domains or subject areas and whatever makes the model different from the rest.
Not only that, but it also covers some Data Management Maturity Models since those also have a data governance component.
Practical Takeaways for Your Data Governance Program
The lessons learned, the key concepts, and the overview of the existing models will provide you with enough knowledge and understanding in order to lead you on the path on how to launch or improve your data governance program.
On-Demand Learning