What Are Some Data Governance Benefits
How will your organization reap rewards from instituting effective data governance? Let us count the ways
- Data consistency means everyone is sharing the same data, no matter where they are in the organization, which prevents a host of operational and risk management issues.
- Data quality improvement means your decisions and operations are running based on accurate and complete information, rather than misleading or outdated data.
- Better decisionmaking thanks to the use of optimized data, so decisions from the C-suite on down can be precise and in touch with actual conditions and operational realities.
- Enhanced business planning as enterprise leaders are able to better project ahead in making key choices.
- Improved financial performance since data governance eliminates errors , and optimizes data-driven decisions and systems so theyre delivering better performance.
Consistently Refine Your Data Governance Framework
Your data governance framework should be a consistent process for data collection and distribution. But, as your business grows and develops, it’s important to adapt your strategy to account for organizational changes. If you don’t update it, you may overlook customer data or accidentally leak sensitive information.
Tips For Creating And Maintaining A Data Governance Framework
Mind the four pillars for success when dealing with a data governance framework, and remember that it is a strategic asset that should be developed, maintained, and protected with care on an ongoing basis. It should be treated as a practice that is embedded in all of an organizations workflows.
Considerations and steps to take when creating a data governance framework include the following.
- Develop a data governance strategy
- Document existing processes, users, and workflows
- Identify where data is, how it is used, and what people or applications use it
- Gather data protection requirements
You May Like: Government Funding For Single Mothers
Why Is A Data Governance Strategy Needed
Theres an old saying that bad facts make bad law. And in the world of analytics, bad data makes for bad decisions. A data governance strategy helps prevent your organization from having bad data and the poor decisions that may result!
Heres why organizations need a governance strategy:
- Makes data available: So people can easily find and use both structured and unstructured data.
- Maintains data consistency: Standardizing data fields across databases and departments makes data easy to manipulate and navigate, .
- Maintains data accuracy: Deleting, updating, or correcting stale or irrelevant data is important to maintain the integrity and value of analytics.
- Supports data security: To pass compliance audits, companies must ensure sensitive data is defined and protected across all locations. This includes where the organization stores, processes, and transmits it
A data governance strategy helps organizations gain greater value from data science and business intelligence tools, as well as the analysts and scientists utilising them. At the same time, it enhances data security and compliance programs.
The Straightforward Guide To Data Governance

Data governance plays a major role in organizing and protecting your internal data. It acts as a form of insurance that every piece of information you collect is properly stored and distributed within your organization.
In this post, let’s discuss what data governance is and how you can implement a policy at your company.
Don’t Miss: Government Personnel Life Insurance Company
What Is Model Governance In Machine Learning
Regulatory compliance Case
Model governance in machine learning comprises multiple areas. The first one revolves around knowing whether your model behaves the way you want them to behave compared to when it was trained. Most models are trained with a limited data set. For example, you might train the data based on a small city in California. When you run that same model for the entire country, it will likely have a different output and decision-making.
So you need some sort of model governance to make sure youre able to capture whether your model has bias, drift, or other performance concerns. This can be particularly important for financial institutions in finance when youre using a model for credit or loan approval where you need to justify why you are rejecting certain demographics. When and if somebody challenges a bank or a regulated industry, they need to provide evidence and an audit report to show they are in regulatory compliance.
Reputation Management Case
The other important aspect to model governance is reputation management. Weve seen cases with social media companies where their algorithm ends up labeling population groups with what looks to the public as racist. Although the people behind the company didnt mean for this to happen, they must still manage the public relations disaster, reputation hit, and loss of substantial profits.
Business Impact
Data Pooling Between Organisations
Data pooling between organisations is the most common alternative data governance model. When organisations, both public and private, hold complementary data , they have an incentive to create data pools. We can distinguish two sub-models in terms of the bundle of rights they create around data: open data pooling and closed data pooling.
It is interesting to point out that both open and closed data pooling are usually initiated by an actor that plays the role of the orchestra conductor i.e. rallying other organisations. This role is typically played by an actor that is legitimate and/or has a tighter relationship with the other organisations pooling data or by the strongest actor among the poolers, as is the case of Airbus in the Airbus Skywise platform.
Read Also: Government Agencies That Protect The Environment
Risks Of Poor Data Governance
While many companies have in-house IT teams, the responsibilities for enterprise data are generally unclear. When there are no clear rules and policies that define who is responsible for what type of data, it creates security loopholes and reduced data quality. With poor data quality, business processes become inefficient, and companies may risk non-compliance with industry regulations. Ineffective data governance processes could cause problems for companies that are required to comply with data privacy laws, such as the California Consumer Privacy Act and GDPR.
With a data governance model in place, a business has clearly defined roles and responsibilities for data management, as well as detailed policies that specify the appropriate processes for collecting, using, storing, and disposing of data in accordance with applicable regulations.
Who Has Ownership Of A Model
Alice: Im working on a project doing X.
Bob: Ah yes, theres some code that does that in Z:/Archive/Misc/Old stuff.
Alice: Theres not many comments in here, can you tell me how it works?
Bob: Dont ask me, I think it was developed by Chris, who left a couple of years before you joined
If your organisation has many people doing data analysis and model building, its important to keep track of who created which models and who is ultimately responsible for their quality and performance. This enables better collaboration, less duplication of work, the enforcement of quality standards, lower risks in dealing with problems and avoids having orphaned models get lost in the ether when someone moves on.
Having a well catalogued inventory of models, plus clear ownership in each case fosters collaboration by allowing people to more easily build on the work of others. Knowing who developed a particular model makes it easier for others to take that work and incorporate it into their own projects. It also gives people an idea of what techniques / domain areas a person specialises in, by looking at the models they have accountability for.
Quality standards are another beneficiary. Compliance with quality guidelines can be included in someones personal objectives. And when new quality standards are imposed, having clear accountability makes it easier to manage and distribute the required efforts.
You May Like: Government Office Furniture For Sale
Centralized Vs Decentralized Data Governance
To understand why data governance is changing, we need to understand the difference between centralized and decentralized data governance models.
Traditional data governance follows a top-down model where business leaders control the master data, and data stewards manage data creation and access points. This gives a central body the authority, responsibility, and control over data access. Larger corporations choose this method to control data by putting limitations and restrictions on specific material.
Decentralized data governance is a newer approach to data governance, arising alongside concepts like data mesh. Decentralized data governance empowers data producers and domain experts to manage and monitor data flow and access points on their own. This model creates multiple groups of authority based on where the data originated.
Types Of Operating Models
Operating models should be unique and specific to the requirements of an organization. While no models fit all, it is important to note that every model should include three polar principles. Below is the difference between the three to help you understand the requirement better:
Centralization Model
A centralized model contains defined standard processes for data governance model implementation. It also determines how data will govern an organization. All teams need to follow defined processes under this model.
Every department can plan and maintain its glossary and data dictionaries in this model. The glossary includes business terms, and data dictionaries contain technical assets. A Federated approach gets followed in-between.
Federation Model
A federation model has multiple authority groups. Organizations need to take it up if multiple teams have different data governance requirements.
Also Check: Right To Petition The Government
What Validation And Approval Is Needed At Each Stage Of Development
As a model passes through the development pipeline, validation and approval should be applied and recorded to ensure a high standard of quality. This reduces the likelihood of any issues making their way through to production, and adds a second layer of accountability.
Validation processes might include:
- Unit testing of feature engineering
- Best practices for Train/Test/Validation datasets and cross-validation.
- Compliance testing
Fundamentally, every model that gets to production should be thoroughly checked and approved by at least one sufficiently qualified person who wasnt directly involved in its development. This compels the developer to justify their decisions and places their work under the scrutiny of fresh eyes.
Benefits Of Operating Model
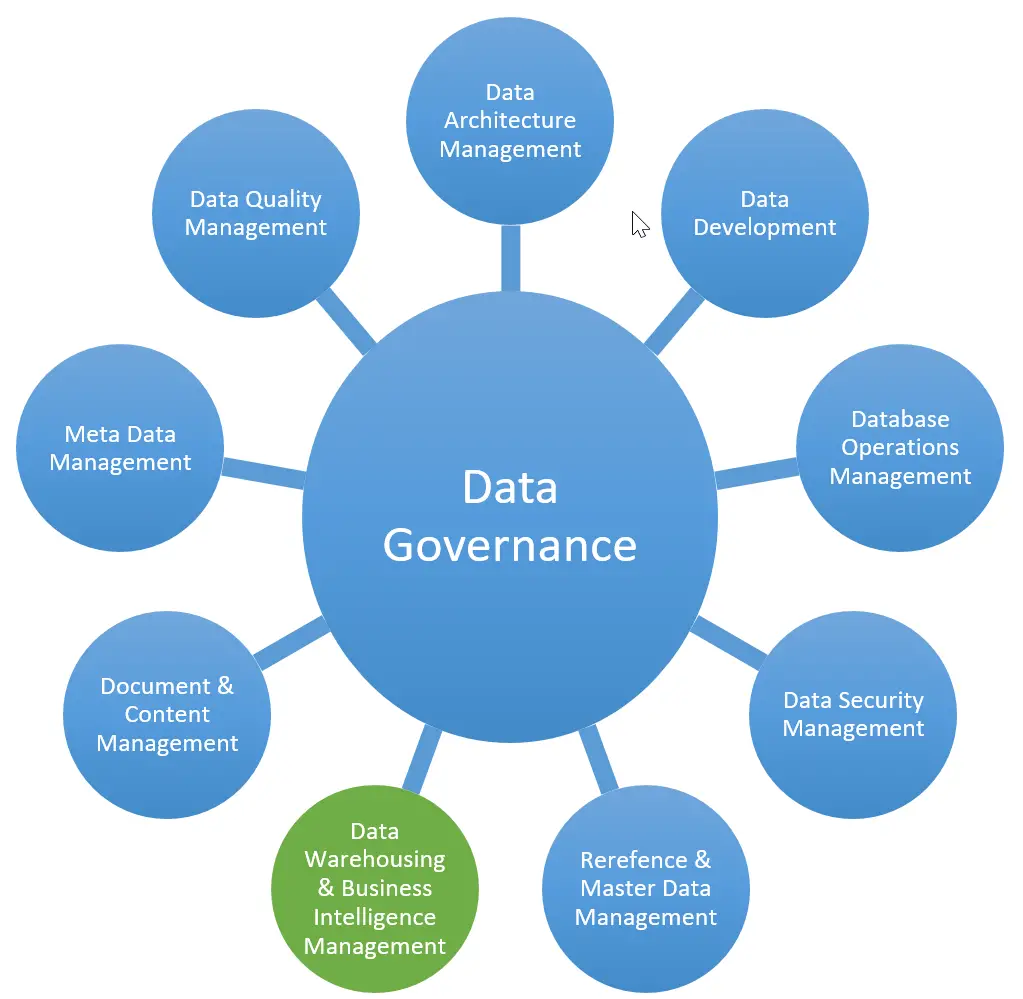
-
Better Clarity: As government principles turn into practices, the operating model addresses the challenge of clearly defining the roles and responsibilities. Not just that, information flow and accountabilities are also analyzed. Collectively, it brings with them better clarity that also helps in decision-making processes.
-
Better Coordination: Operating model requires addressing the complexities of the challenges. The process requires better coordination to tackle it. It also helps to strike a balance between important business considerations.
-
More Visibility: With an operating model, there is better visibility of management, its decision-making powers, and risk processes.
-
Practical Approach: The model will help the organization carry out duties in a more reliable manner. It also helps in sound data governance.
A well-planned operating model acts like a bank to store inventory processes that help overcome the gaps during policy and implementation processes. Data governance professionals play a significant role in identifying and planning it.
Also Check: Data Governance Council Roles And Responsibilities
Creating Your Data Governance Framework
Once youve answered the questions above and settled on your team, youll have a solid foundation on which to build a data governance framework. But in building it, you cant proceed randomly theres a logical progression of steps you need to follow.
Determine your data governance strategy
Create a data strategy by bringing together existing processes, people, and workflows.
Start small
Start with just one business area or data issue and expand from there. Hint: Its good to start with one where significant problems or imminent risks make it a good testing ground for data governance.
Pick the right framework
Continue to make refinements to your framework as your business needs change.
Data Governance Vs Data Management: Are These The Same
Data governance is not the same as data management.
For example, if we are to compare data with customers, front-line customer service agents implement a company’s customer service policies, while the customer service leadership team designs the strategies, policies, and processes. Implementation flows from having a clear strategy, processes, systems, policies, and procedures.
The same applies to data governance. Data management is the practical implementation of data governance principles, and is primarily seen as a function of an IT department. Data management encompasses the lifecycle of a company’s data assets.
Whereas, data governance is the creation of a set of standards, principles, processes and systems that oversee the management of data within an organization.
With an effective data governance model, organizations experience numerous benefits, including:
- Ensuring data consistency across an organization, and that data is trustworthy and doesn’t get misused
- Managing and navigating data compliance and privacy laws worldwide
- Breaking data out of silos so that it adds more value across an organization
- Supporting leadership and front-line teams with more effective access to data to help optimize operations and drive data-driven decision-making
Business leaders make better decisions when they have access to the right data, at the right time, in a format they can use. An effective data governance model founded on the principles of data governance makes this possible.
Read Also: Government Personal Loan For Private Employees
What To Look For In An Ai Governance Solution
The best AI model governance solutions focus on simplifying the entire process of monitoring and compliance assurance, while not causing significant disruptions to existing workflows and allowing key stakeholders in the organizations to have visibility into what the models are doing.
Most solutions today try to focus on how to help the data scientist figure out whether models are running correctly in the lab environment with a limited training data set. Furthermore, it requires specific integration that adds to the data scientists workflow.
It is also critical to use a governance tool that is agnostic to the model development platform, thereby empowering data scientists to develop best-in-class models using the right ML development platform. The ML world is too nascent there is no such thing as a end-to-end platform that can handle the entire lifecycle of development to deployment. Enterprises need to be cautious not to buy into the marketing nonsense and only find themselves locked into an overpriced tool that brings no benefits. Even worse, it further creates dissolution in the enterprises that AI projects are a waste of money.
However, what is more relevant and helpful, is measuring in a production environment without interfering with or tapping into what the data scientists are doing. The best way to think of this question is in layers of defense.
What Is Model Governance And Why Its Important
Businesses including financial institutions, pharmaceuticals, manufacturing, and more rely on data scientists to create models to improve specific functions of the business, like fraud prevention. Once these models leave the lab environment, theres very little visibility on what happens to them. Questions like, How are the models being deployed? and Are the models making the right decisions? and Are there biases? must be answered.
During development, machine learning models are bound by specific assumptions, rules, and expectations. Once the models are deployed, the results in the real world can differ significantly from the results in controlled development environments.
Having good documentation showing the lifecycle is useful but insufficient when models become unreliable. AI model governance brings accountability and traceability to machine learning models they ask and give answers to these crucial questions:
- What input variables are coming into the model?
- What are the output variables?
- What were the previous versions like?
- Who has access to the model?
- Has there been any unauthorized access?
- How is the model behaving with certain metrics?
Recommended Reading: Loans For Government Employees With Bad Credit
Phases Of Building An Operating Model
After defining the model requirement, there is a need to understand the best practices suited for each organization. Before launching a data governance practice, key elements of the journey should get planned in three phases. Find how Royal Cyber’s Data Governance experts can help you.
Defining the Processes
The operating model process is important to define in the beginning. It should start from assigning roles and responsibilities as well as information assets. Data catalog, glossaries, and dictionaries should also get defined in the beginning. The final step should illustrate control measurement. To simplify it, the three areas of focus should be around roles, framework, and stakeholders.
Identification of Data Domains & Critical Data
The next step in the process is the identification of data domains and critical data within the domain. Think of the data governance process as a bridge between people, processes, and technology. The need to identify data domains springs with the need to resolve a problem or drive business opportunities. Each organization has a different set of challenges and opportunities. It is important to define the initial processes to streamline the result. Data governance experts can help you narrow the focus of your data governance program. Consult a Royal Cyber expert today.
Design and Reporting Structure
Role Of Data Governance
The main role of data governance is to ensure that the data quality remains high throughout the complete lifecycle of the data and the controls which are implemented are in line with the organizations business objectives. It is important that information is used both effectively and efficiently and is in line with the companys intentions. Data governance identifies who can take what action, as a result of what data, in which situations, and using what methods.
You May Like: Free Government Phones In North Carolina